Adjust instruction based on real-time student data
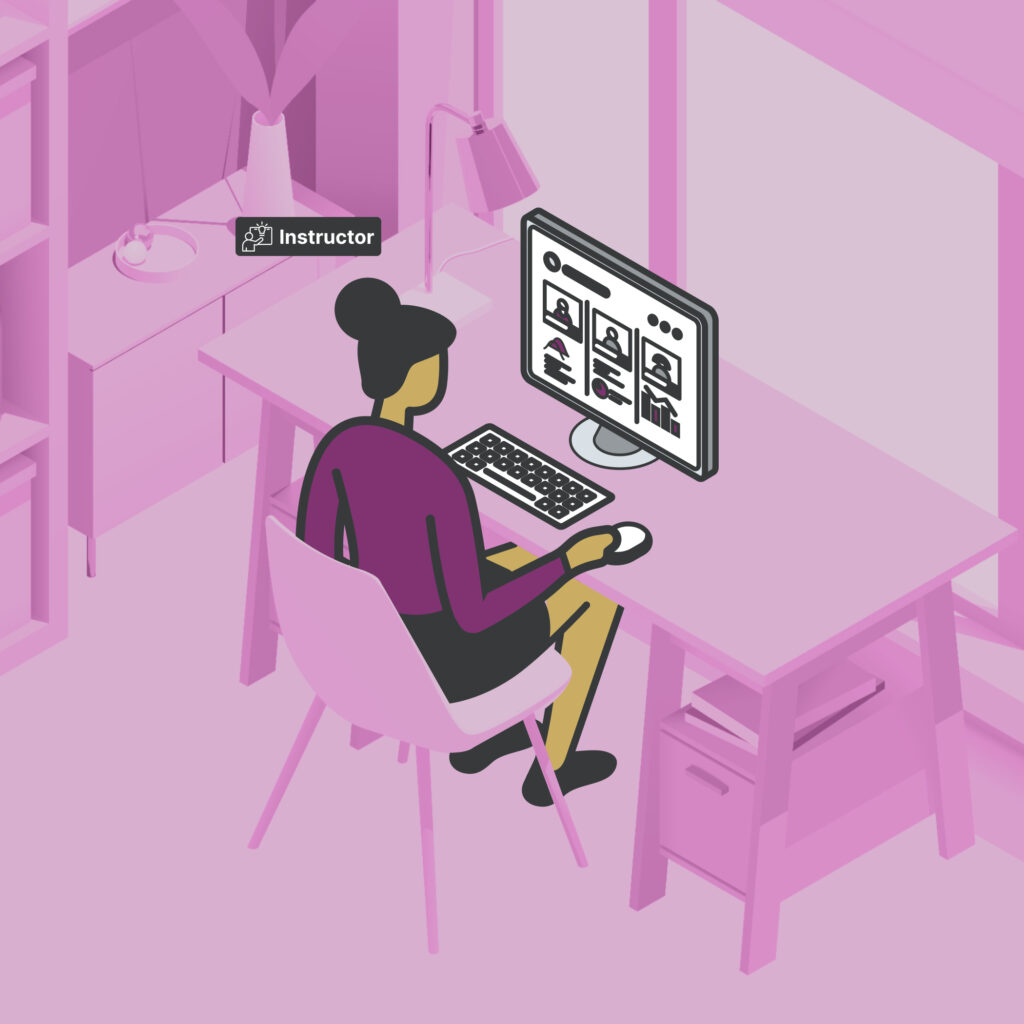
Data-informed instruction calls on professors to use real-time, disaggregated data to inform proactive practices, allowing them to identify challenges quickly and make adjustments at the class level throughout the duration of the course.
Professors can also use student learning data to provide personalized and differentiated instructional pathways for students. By leveraging this practice, professors enable students to analyze their own course-specific data to understand their learning habits and practice metacognition, track progress toward learning goals, and receive just-in-time feedback on assessments.
How Data-Informed Instruction can be Digitally-Enabled
The ability to manually collect real-time data and effectively enlist that data to inform instructional choices is challenging even in small enrollment courses much less large enrollment courses. Data-informed instruction benefits tremendously when high-impact data points do not have to be intentionally collected by instructors but instead can be generated from instructors’ and students’ normal day-to-day interactions with digital teaching and learning tools. Digital assessments and curriculum tools can support proactive adjustments to instruction by generating critical data points and analyses with more efficiency and accuracy than humans alone can. Furthermore, these tools can support instructors, departments, and institutions to identify inequitable disparities in student learning outcomes in courses by leveraging additional data around ethnicity, race, gender, native language, and other demographic factors.
Key Dimensions of Data-Informed Instruction
Collection of real-time data
Professors continuously collect real-time data to inform their instruction, which may include data on student interactions with courseware products, assignment completion, and engagement metrics. Professors gain a dynamic and current snapshot of student performance and participation throughout the course.
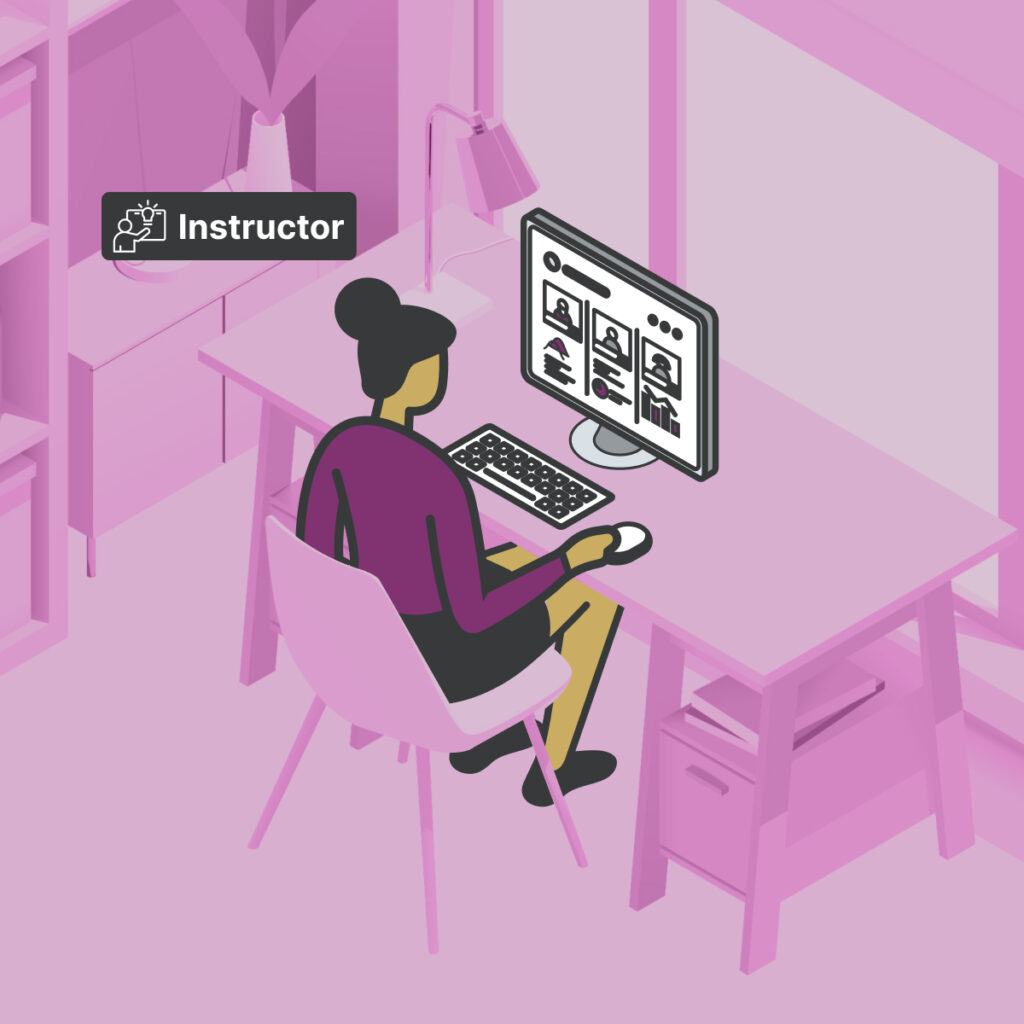
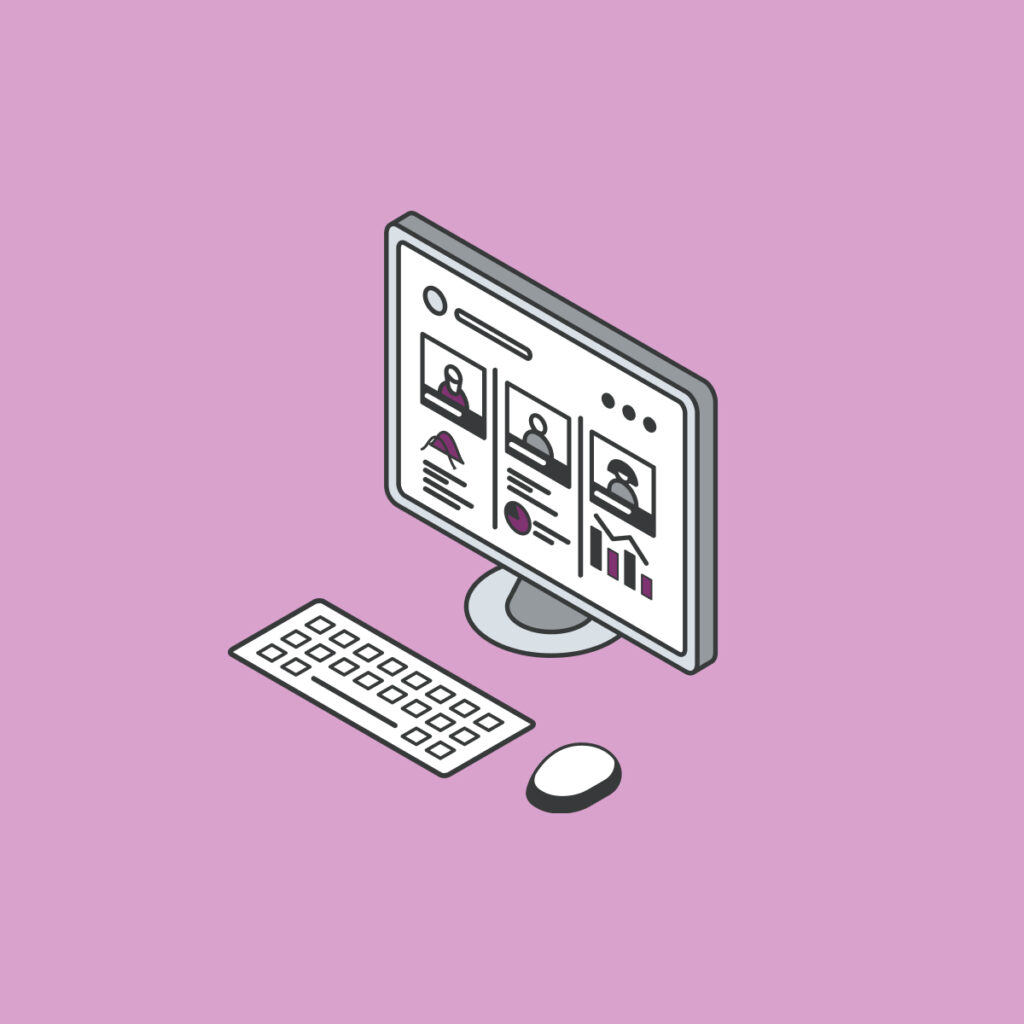
Disaggregated data analysis
Professors engage in a thorough analysis of the collected data, breaking it down by ethnicity, race, gender, native language, and other factors, to gain insights into individual and group performance. This disaggregated data analysis enables professors to better understand the needs of their students, tailor interventions to address specific challenges, and promote equitable learning outcomes.
Proactive interventions and adjustments
Informed by insights from data analysis, professors proactively intervene to address challenges and make timely adjustments to their teaching strategies. They respond to student needs promptly, fostering a more supportive and responsive learning environment.
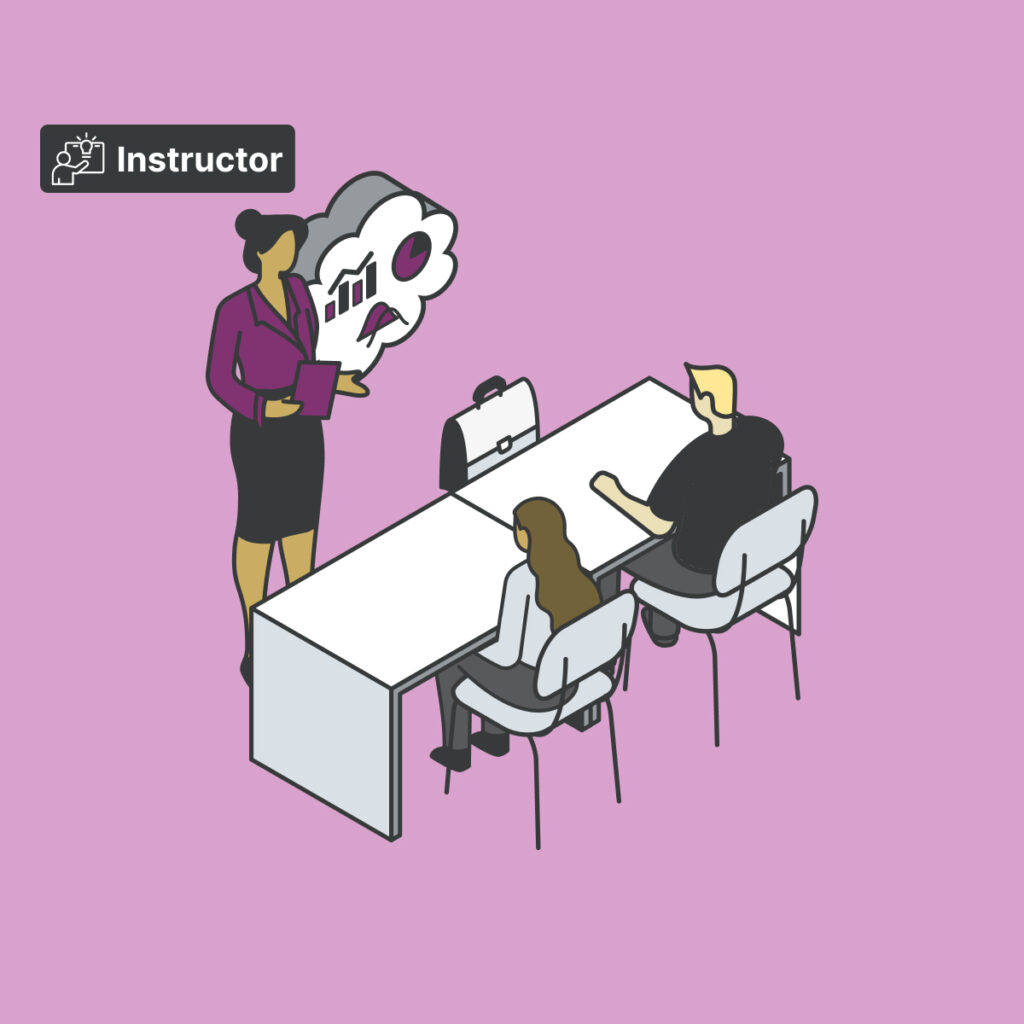
Instructional Examples
The Instructional Example Library features a wide range of digitally-enabled examples sourced directly from instructors who are using technology to implement evidence-based teaching practices in their courses. These examples focus primarily on math, chemistry, and statistics gateway courses, but are applicable across disciplines.
Have an Example of Your Own?
Help us build our Instructional Example Library! We are looking for contributions from higher education instructors across disciplines who use technology to enable evidence-based teaching practices. To learn more and to submit an example, please visit the form page linked below. Thank you for helping us support the field.
Further Reading
- “Disaggregating Learning Data to Support Equity Efforts,” written by Every Learner Everywhere, offers professors a guide to gather and effectively act upon disaggregated data throughout their courses.